Theta Informatics LLC
The ability to locate any point within images taken by common off the shelf drones has been demonstrated by OpenAthena™ software published by Theta Informatics. This is free and open source software that combines sensor metadata embedded in drone images with publicly-available digital elevation models of terrain to determine the precise ground location of any pixel within a drone’s images.
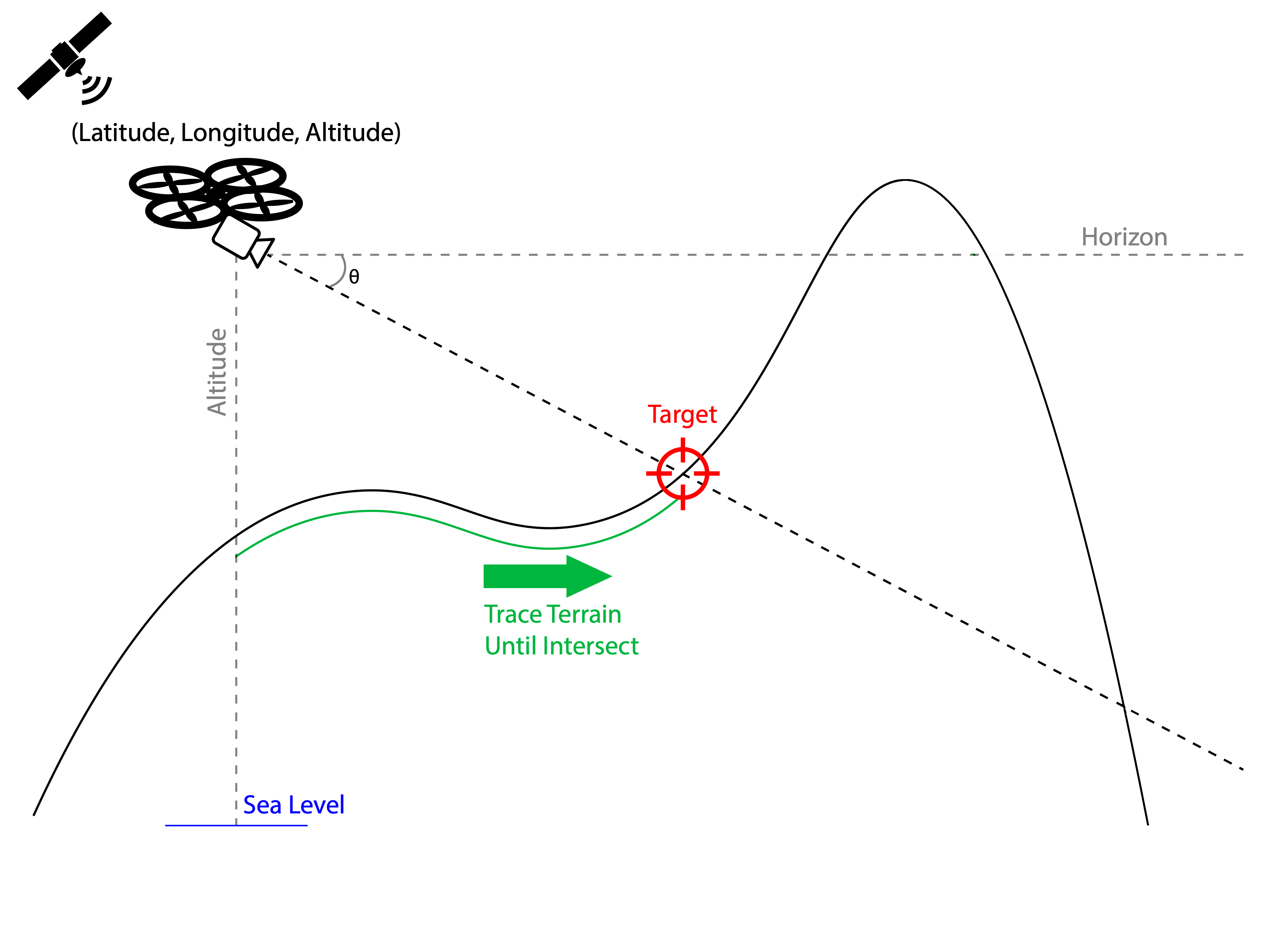
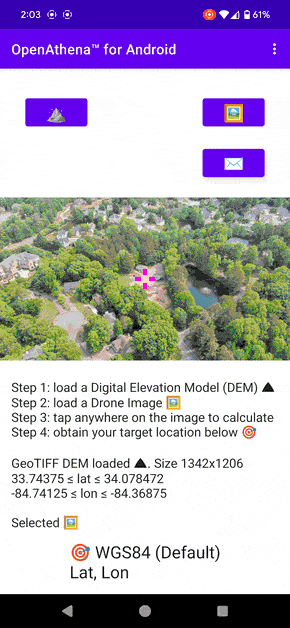
While this software is dual use (made for both commercial and defense applications), we have begun development of new AI object detection models for defense applications. Such models are capable of automating the process of finding military objects within drone images and locating them. This technology is anticipated to be highly disruptive to conventional maneuver warfare doctrine. These models will be proprietary, closed source products, not for export until their full compliance with U.S. ITAR law is attained.
Theta Informatics has developed an AI object detection convolutional neural network model named “Naissance”. Based on a variant of the YOLO object detection framework, it has been trained on an extensive dataset of real world, synthetically generated, and hand-labeled training images.
The first iteration of Naissance is trained to recognize just one object class: The Russian T-72 Main Battle Tank.
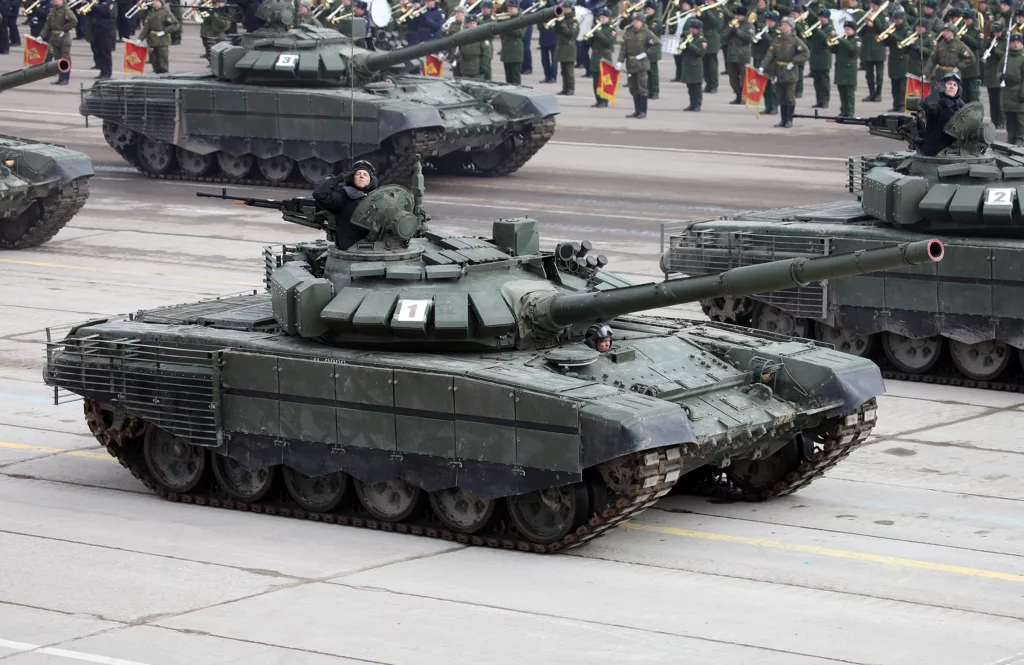
On a validation test performed with 932 images never seen by the model during training, Naissance demonstrated significant precision and accuracy:
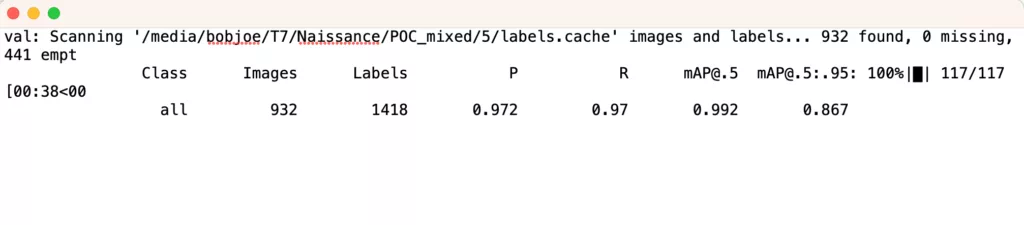
In this test, the model attained a precision statistic of 97.2%, indicating that false positives only occurred for 2.8% of detected objects. It had a recall statistic of 97%, indicating only 3% of objects were ever missed by the detector.
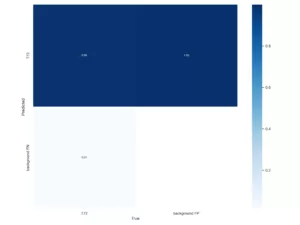
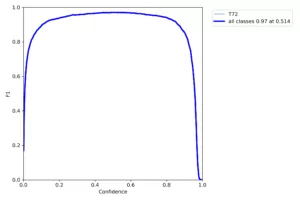
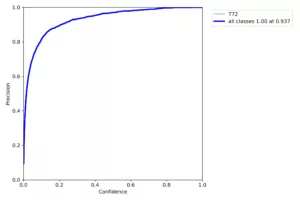
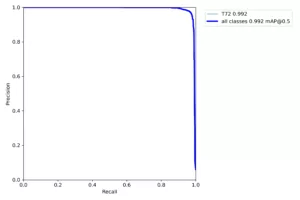
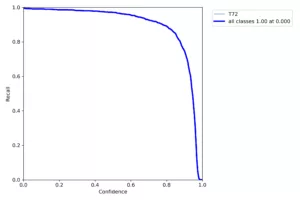
The dataset for training this model was a mix of synthetic computer graphics renderings and real images of a T-72 MBT in a multitude of authentic contextual settings for the military domain. Drone images of real world scenes, without objects present, were used as negative data to improve the model’s accuracy and reduce its rate of false positives in a variety of conditions.
The dataset for this model was labeled by U.S. based Theta Informatics employees by hand, over 41.5 hours across 31 sessions of labor.
The availability of high quality, diverse, and accurately-labeled training data is the primary concern for creation of high quality object detection models. Models trained with a higher volume of representative data are more robust under a variety of conditions.
-Matthew Krupczak
CEO, UAS Geodesist
Theta Informatics LLC
To see more from Theta, please follow our official X (formerly Twitter), YouTube, and LinkedIn pages.
One response to “Press Release – Naissance AI Model Prototype for detection of the T-72 Main Battle Tank”
[…] a previous blog post, we discussed some recent success Theta has achieved with our prototype AI model named […]